When I was asked to lead the Intelligent Systems and Technology Lab at the Palo Alto Research Center (PARC), I wanted the lab to succeed wonderfully in its research mission. I was a fairly prolific inventor and had taken multiple tours of duty as a research manager. I set out to open my mind to possibilities for effective invention and innovation by interviewing colleagues at PARC and elsewhere about their best practices.
How do you create the conditions under which inventions arise?
I shared insights from the inventor interviews with my wife, Barbara. Soon, she had her own recording device. She joined me on the interviews and did additional ones on her own. Three years later our work led to the publication of our book Breakthrough by The MIT Press.
One of the most interesting questions that we asked and sought to answer was “How do you create the conditions under which inventions arise?” We also asked “How do you create the conditions under which innovations arise?” The distinction is that invention is about developing ideas and innovation is about developing inventions to become products.
Today there are needs and opportunities not only for inventing and innovating, but also for new technologies and ways of inventing and innovating.
It is timely to ask these questions again. Today there are needs and opportunity not only for invention and innovation, but also for new technologies and ways of inventing and innovating.
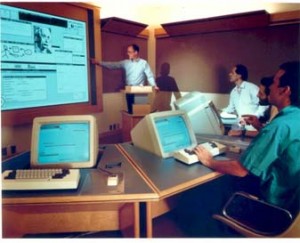
PARC’s Colab (1989)
When I came to PARC, I loved the wall-sized whiteboards. We developed group processes of gathering at a whiteboard, brainstorming, and organizing our ideas. I wanted to speed up the process for rearranging the drawings and symbols on the whiteboard in our intense idea sessions. This led to a DARPA-funded Colab project where we created an electronic meeting room, including a wall-sized projected screen made touch-sensitive by special hardware from the Xerox skunk works.
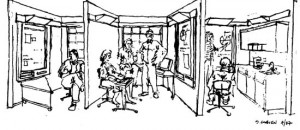
Portable meetings and portable ideas were intermediated by interactive whiteboards
We invented WYSIWIS (What You See Is What I See) interfaces and public and shared windows. Our team productivity was accelerated by conversations that combined the familiar one-person-at-a-time audio channel of meetings with computer-intermediated visual elements. The tools also created digital outlines and other records of our work as described in our paper.
Our multi-user Colab tools worked best when we were in the Colab. John Seeley Brown and I saw a possibility (not then technically practical) for creating meetings and idea-structures that were “portable.” They could be resumed, rewound, re-branched, and extended. Our paper “Towards Portable Ideas” imagined how strategically-located interactive whiteboards with a suitable infrastructure could extend the utility and life of conversations as “portable ideas” were created, carried and refined by work groups. This idea on boards, tablets, and other devices is ripe to be realized today.
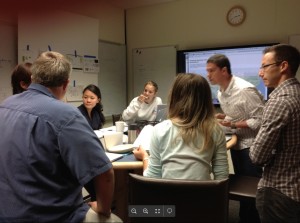
Design Lab created for the Technology for Agile Organizations (TAO) group at PARC
Colab technology led to a Liveworks PARC spin-out with a “LiveBoard” product, which was expensive at the time. Many years later, using off-the-shelf technology, we built a simpler design lab for stand-up meetings and working design sessions for a smart cities project. Meetings were BYOC (Bring Your Own Computer) and seating was at a stand-up table to encourage high energy interactions.
How can we better support opportunity awareness and powerful conversations for innovation in distributed organizations?
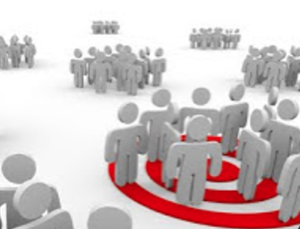
How could a virtual workplace support serendipitous interactions?
Forward to the COVID pandemic. Many workers had completed a year of desk-bound remote working. They practiced sharing screens in online meetings, having targeted side conversations with text messaging, and finding the mute button.
The pandemic has probably accelerated the shift of organizations to geographically distributed forms where teams work at different sites, and more employees work remotely.
But are the remote workers and teams as effective at invention and innovation? Are distributed teams and teams of teams less-powerfully connected and less effective? Are important opportunities being missed?
Despite long hours in meetings, it seemed harder to have the formal and informal conversations afforded by physical workplaces where ideas are seeded and developed.
The pandemic has probably accelerated the shift of organizations to geographically distributed forms, where teams work at different sites, and more employees work remotely.
A physical workplace offers affordances for informal interactions. Meetings around problem solving or idea development are open and attract opportunistic engagement. Consider a physical office workplace as in the picture. What are its affordances for:
- Sound boarding ideas
- Learning about new activities (noticing the “buzz”)
- Catching up quickly on progress
- Creating partnerships
- Onboarding new team members
Current online meeting software is focused on scheduled meetings. The meetings are often not serendipitous, engaging, or effective. Management has little visibility into collaborative engagement opportunities in the virtual workplace. It can not easily see how well today’s remote meeting tools promote collaboration and coordination. People talk about burn out from on-screen meetings all day long.
Especially in regions like Silicon Valley, the limited available land area has led to a dramatic rise in the cost of real estate. This cost creates substantial overhead for businesses and also challenges for new employees who want to buy homes and raise families. In this way, the local concentration of technology development is itself creating economic pressure for policies to allow remote workers. Do we need a new generation of infrastructure for distributed organizations?
Current online meeting software focuses on scheduled meetings. The meetings are often not serendipitous, engaging or effective.
Forward to today. I am the principal investigator of the COGLE (Common Ground Learning and Explanation) project. COGLE is part of the Defense Advanced Research Projects Agency (DARPA) eXplainable AI program. It is supported under contract FA8650-17-C-7710.
In writing up findings of a user study about XAI explanation I found myself reflecting on the Stanford DENDRAL project, which routinely solved complex chemical structure elucidation problems. DENDRAL had superhuman performance in solving chemical structure puzzles. Carl Djerassi, a Stanford chemistry professor and inventor of the birth control pill, was one of the leaders of the project. In his autobiography, Djerassi reported on a pedagogical experiment. He assigned his students in a graduate chemistry seminar to use DENDRAL to check the chemical structures that were reported in papers from peer reviewed chemistry journals. Without exception they found that every article had mistakes. The articles presented evidence and identified the chemical structures that were consistent with it. For every article, there was at least one structural alternative that the authors and reviewers had missed. The point is that combinatorial problems are difficult to get right, even when people check each other’s work.
COGLE’s domain is about autonomous drones that carry provisions in mountainous and forested settings to stranded or injured hikers. The connection here is that like DENDRAL, COGLE’s AIs have superhuman capabilities. They find optimal flight plans for missions in a simulation world. One configuration of COGLE is as a decision aid for humans, who choose the best AI-controlled drone for a mission. The AIs at hand have different experiences, know different things, and make different plans.
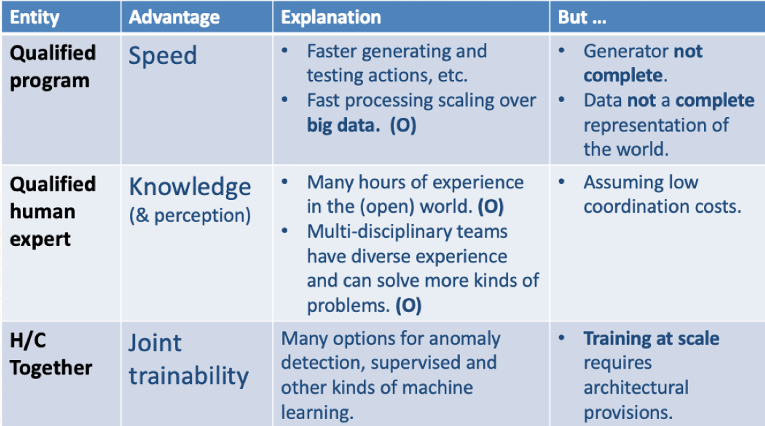
Typical advantages of human and AI cognition on combinatorial problems in open (O) worlds.
Although COGLE’s AIs are great optimizers, they are limited by the specific experiences in their individual training. The different AIs have different experiences and knowledge. User study participants had to judge when they should trust an AI and when they should not. Sometimes the human — or even the Explainer — can tell that the AI-generated plans have issues.
This application example frames one of the important uses for XAI technology: decision support. People and computers have different advantages and can complement each other on a human plus computer team. There are other cognitive asymmetries and applications as well and studies to understand where and when AIs can best complement humans. Matt Johnson and Alonso Vera make the case that the greater the power of an AI on a team, the greater is its need for skills for collaborating with people. Teaming intelligence is all about team members understanding, supporting, and exploring their interdependence in the partnership.
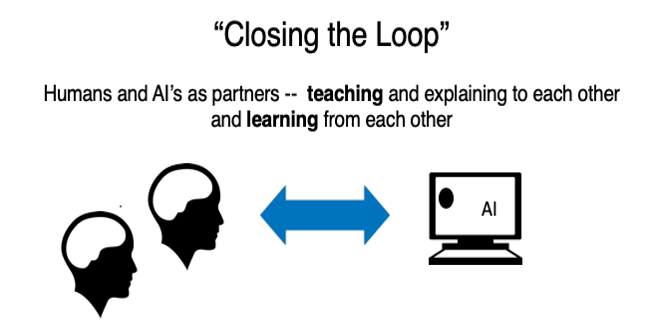
Closing the Loop refers to AI systems that can both explain and take advice.
At a principal investigator meeting of the XAI program I was asked to lead a panel about a research area beyond XAI called “Closing the Loop” (CTL). CTL casts XAI as half of a solution. An XAI has deep or other form of machine learning augmented by explanation capabilities. In the other half of a CTL, the AI takes advice and collaborates with people. Ideally, a CTL system can participate in a conversation with users, develop common ground with them, improve knowledge and performance over time, and be a collaborative computational partner. Another technical term for this idea is Interactive Task Learning (ITL).
The panel also considered the DARPA-hard research challenges in creating CTLs. Like XAI, CTL requires multi-disciplinary perspectives to create the AI and to understand the requirements for effective human plus computer teaming.
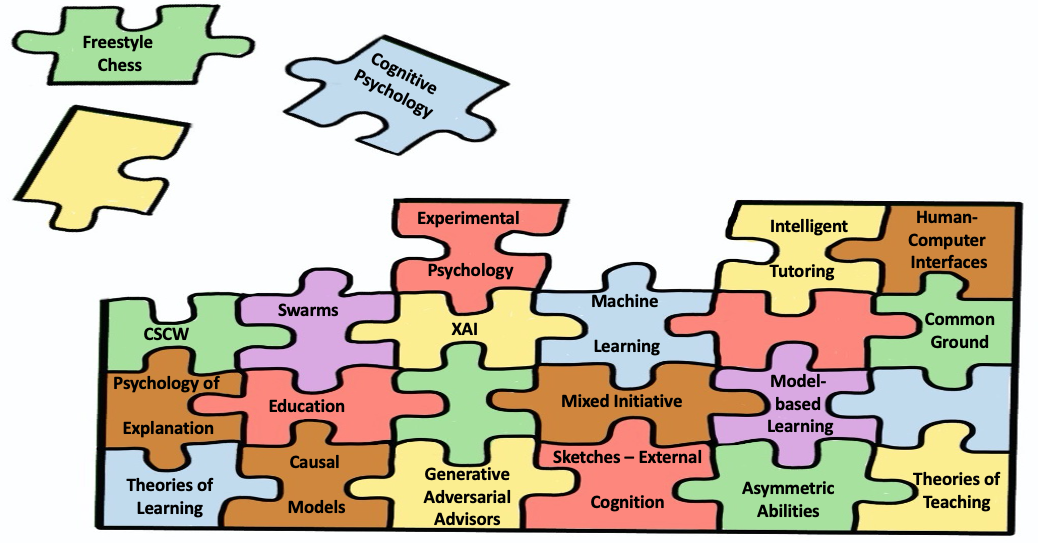
Disciplines and ideas as pieces for a CTL project.
In his bestseller book Leading Matters, former Stanford University president John Hennessy offered his thoughts on the nature of society’s big challenges — which include such issues as responding to the pandemic, energy, the fragile environment, and others. Such problems do not fall within single disciplines or have easy fixes. They are multi-dimensional and interdisciplinary. Even developing an understanding of such a problem does not fit easily in one person’s mind or their education and experiences. Shared purpose, collaboration and determination are required.
Multi-disciplinary teaming is required to address many of today’s big challenges. Teams that include AIs as partners may become essential — even the norm.
A recent call for action by the National Security Commission on Artificial Intelligence, (Final Report) advances a case for a large and sustained national effort for research and development of AI science and technology. This is another piece of an answer for our opening question.
This post started with the question “How do you create the conditions under which innovation arises?” In the years since our Breakthrough book was published, several things have happened. The world’s problems have become harder, and the technology has advanced. Our unaugmented human minds have not advanced.
Revisiting the question a decade and a half later, we see new opportunity in how to plan our collective journey. The journey involves new tools to support human collaboration in distributed organizations. It also includes developing AI partners to join the team.
Many thanks to Edward Feigenbaum and PARC colleagues for conversations in developing these ideas.
Post by Mark Stefik with contributions from Michael Youngblood